Data is everywhere. From point-of-sale information collected by retailers to traffic sensors, satellite imagery and the daily tidal wave of social media posts, we produce billions of gigabytes worth of information each day.
U of T Engineering researchers are drawing critical insight and information from mass data by marrying emerging techniques in big data, deep learning, neural networks and artificial intelligence (AI) to design smarter systems. We are creating algorithms that crawl through the genome to understand how specific mutations result in diseases such as autism, cancer and cystic fibrosis, and suggest possible treatments. We are teaching computers to recognize speech and images, including individual human faces, with applications in sectors from security to aesthetics. We are also building computer models that optimize surgical schedules, making better use of valuable resources.
Society is in the midst of what is being called the Fourth Industrial Revolution. Data analytics and AI have tipped the balance, fundamentally changing the way we do business, treat disease, interact with technology and communicate with each other. Our expertise in these areas will help to reshape processes to improve lives and generate value for people around the world.
RESEARCH CENTRES & INSTITUTES
-
2019: CENTRE FOR ANALYTICS AND ARTIFICIAL INTELLIGENCE ENGINEERING (CARTE)
CARTE is advancing engineering-focused analytics and AI research and translating the associated technologies into real-world impact. CARTE aims to cultivate industry partnerships, drive collaborative research between AI experts and those with domain-specific knowledge, and help meet the surging demand for engineers who are well-trained in this field.
-
2017: Vector Institute
Building on the existing expertise of the globally renowned deep learning team at U of T, the Vector Institute is driving excellence and leadership in AI to generate talent, foster economic growth and improves lives. The Institute is funded by the governments of Ontario and Canada, and collaborates with 30 industrial partners, including Google, Uber, Shopify and several financial service institutions. Read more about the Vector Institute.
-
2017: Computer Hardware for Emerging Sensory Applications (COHESA)
This NSERC Strategic Partnership Network brings together researchers from academia and industry to design computer hardware optimized for applications in machine learning and artificial intelligence. Industrial partners include chip manufacturers AMD and Intel as well as technology firms such as Google, IBM and Microsoft. Read more about COHESA.
-
2012: Southern Ontario Smart Computing Innovation Platform (SOSCIP)
SOSCIP is a research and development consortium that pairs academic and industry researchers with advanced computing tools to fuel Canadian innovation within the areas of agile computing, cities, mining, health, digital media, energy, cybersecurity, water and advanced manufacturing. Read more about SOSCIP.
-
2011: Smart Applications on Virtual Infrastructure (SAVI)
This NSERC Strategic Partnership Network built a next-generation internet platform that investigates cloud computing, software defined networking and Internet of Things. One use case is Connected Vehicles and Smart Transportation, which integrates data from a wide variety of sensors — highway cameras, road incident alerts, transit systems, border crossings — to help ease congestion, improve personal safety, increase energy efficiency and reduce waste in smart cities. Read more about SAVI.
-
1991: Centre for Management of Technology and Entrepreneurship (CMTE)
CMTE creates innovative solutions within the Canadian financial services industry in three main areas: financial modelling, data mining and analytics, and machine learning. This multidisciplinary centre also provides engineering students with industry-focused learning opportunities to further develop talent in these areas.
EXPERTISE
Selected areas of expertise in data analytics & AI research at U of T Engineering
- Artificial Intelligence
- Augmented Reality
- Autonomous Vehicles
- Bioinformatics
- Communications
- Computing Systems (from Internet of Things to hardware and software)
- Computer Architecture
- Computational Medicine
- Cybersecurity
- Data Mining
- Fintech
- Intelligent Robotic Systems
- Intelligent Transportation
- Machine Learning
- Neural Networks
- Smart City Platforms
RESEARCH IMPACT
An Artificial Intelligence Innovation HQ in Toronto
For artificial intelligence researchers at U of T, starting a new industry collaboration is as simple as crossing the street.
In the summer of 2018, the LG Electronics AI Research Lab was launched, along with a five-year, multi-million dollar research partnership between LG and U of T. Located right next to the St. George campus, the AI Research Lab is catalyzing more than a dozen collaborations between employees of the company and U of T researchers in both engineering and computer science.
The partnership enables LG to continue improving the functionality of its products, from TVs to smartphones, while accelerating U of T's fundamental research into new AI algorithms and approaches. Potential applications include autonomous vehicles, data driven protection, and supply chain management.
Through ‘reverse internships,’ LG staff scientists spend up to four months working in academic research labs returning to the company better equipped to tackle more challenging problems. There is also the ‘mini-master’s degree,’ in which researchers from LG’s primary labs in South Korea travel to Toronto to work alongside U of T Engineering professors to refine their skills by completing a capstone project.
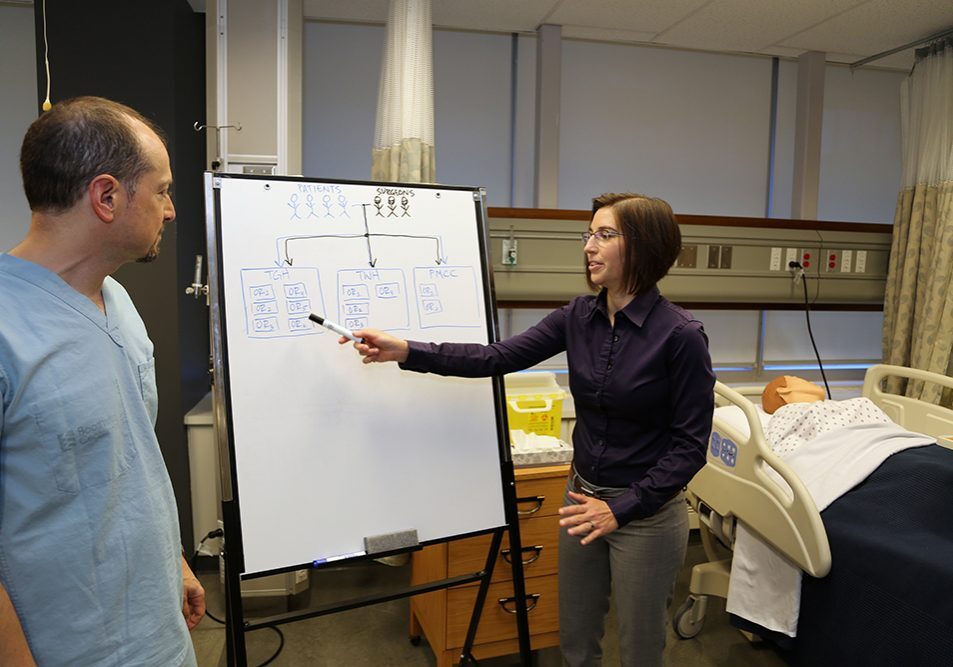
Optimization for smarter health care
Long waitlists for elective surgeries are a major challenge in the Canadian health-care system. According to industrial engineering professor Dionne Aleman (right), the problem may not necessarily be a lack of resources, but rather a result of not using the resources we have as efficiently as we could.
As a member of the Centre for Healthcare Engineering at U of T, Aleman collaborates with medical professionals such as Dr. David Urbach (left), a surgeon and senior scientist at the Toronto General Research Institute, on a variety of issues in the delivery of health care. Using data from Toronto General Hospital, Toronto Western Hospital and the Princess Margaret Cancer Centre, Aleman and her team have built a mathematical model that can optimize the matches between patients, surgeons and operating rooms to generate the most efficient schedule.
One technique the team uses involves pooling resources. Rather than each hospital maintaining its own waiting list, patients would be treated as a single large waiting list, and assigned to a given surgeon or operating room to minimize the time when resources are unused. Using this approach, Aleman and her team have shown that they can increase the number of patients treated in a given time period by up to 30 per cent.
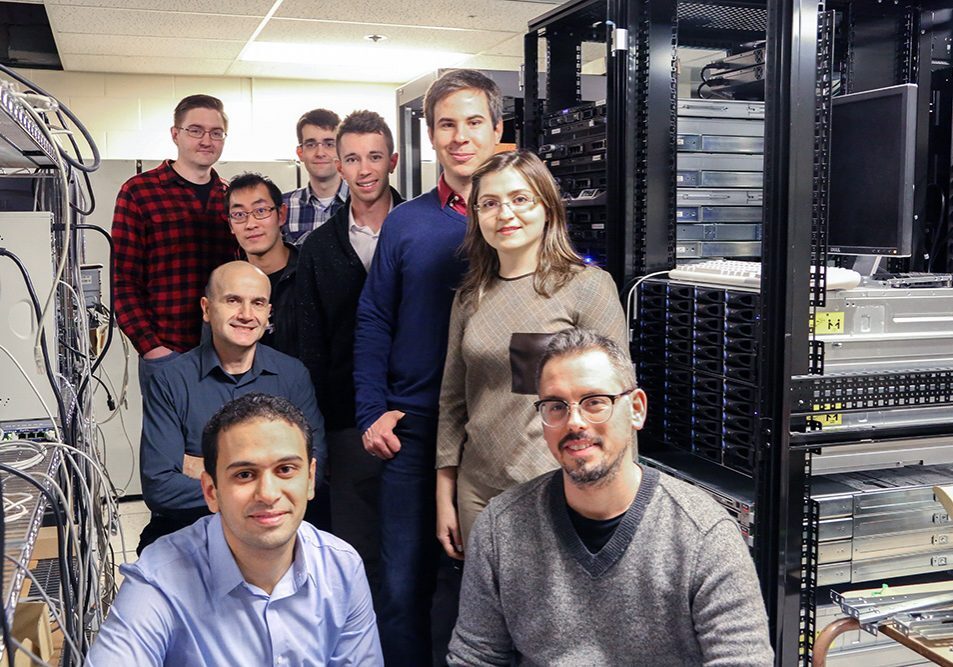
Hardware acceleration for deep learning
Since the 1970s, the number of components that can be fit into an integrated circuit has doubled roughly every two years, a phenomenon known as Moore’s Law. Faster processors have fueled the rise of machine learning and artificial intelligence, but as these applications become more widespread, the need for speed will only continue to grow.
Professor Andreas Moshovos (middle row, left) in The Edward S. Rogers Sr. Department of Electrical & Computer Engineering and his team design computer chips that are optimized for machine learning applications. Just as graphics accelerator chips improve video displays by completing many common operations in parallel, chips optimized to execute machine learning calculations can offer speeds anywhere from 10 to 1,000 times faster than general-purpose processors.
Moshovos heads NSERC COHESA (Computer Hardware for Emerging Sensory Applications), a national network of researchers from academia and industry that are finding ways to eliminate needless or repetitive operations and create chips ideally suited to artificial intelligence. In the future, such chips could power smarter voice-activated assistants, video recognition software or self-driving vehicles.
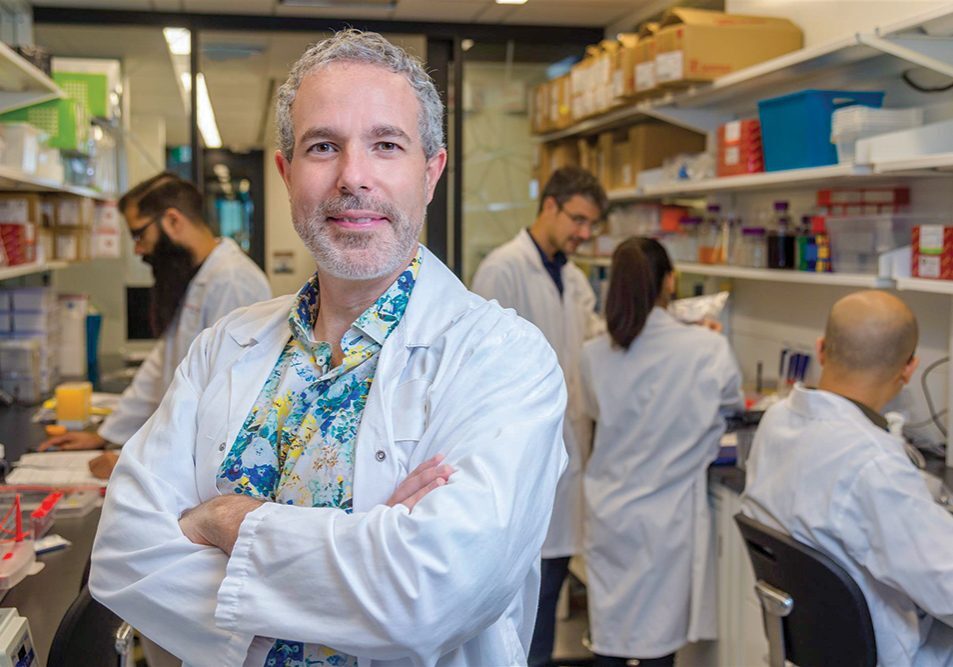
Mining genomic data
The Human Genome Project began in 1990 and took more than a decade and billions of dollars to complete. Today, a genome can be sequenced in an afternoon, for a few hundred dollars. The key challenges of genomics lie not in generating the data, but in transforming that data into useful knowledge.
Deep Genomics, founded in 2015 by electrical and computer engineering professor Brendan Frey, Canada Research Chair in Information Processing and Machine Learning, is on a mission to predict the consequences of genomic changes by developing new deep learning technologies. The company grew out of Frey’s research on deep learning, a form of artificial intelligence that has already revolutionized web search, voice recognition and other areas. By applying these techniques to gene sequence data, Frey and his team aim to not only understand how mutations lead to diseases such as cancer, cystic fibrosis and autism, but also point the way toward drugs or other interventions that could better treat these conditions.
Deep Genomics has raised more than $20 million in funding and aims to double its staff levels from 20 to 40 employees in the near future.
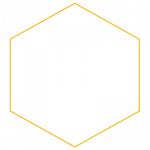
“Humans and machines think differently, and they excel at different tasks. Our job as software and hardware engineers is to design machines and algorithms that perform their tasks faster and more efficiently, which frees up human capital to focus on creativity and innovation.”
PROFESSOR NATALIE ENRIGHT JERGER
Percy Edward Hart Professor of Electrical
and Computer Engineering
THE FUTURE OF DATA ANALYTICS & AI
A global hub for AI engineering
Artificial intelligence has deep roots at the University of Toronto. Yet increasingly, there is a critical need to leverage advanced analytics and AI tools for insight generation and improved decision making across all industries. Founded in 2019, the Centre for Analytics and AI Engineering (CARTE) aims to facilitate the translation and application of AI research, technologies and solutions to a range of industrial sectors.
Led by Professor TIMOTHY CHAN, CARTE is organized around three core pillars: research; education and training; and partnerships. CARTE will play an active role in cultivating demand from industry, enabling collaboration and accelerating the education of AI-savvy engineers.
Improving network agility through softwarization and intelligence
In Canada, the major internet service providers began life as telecommunications companies. As they shifted from providing telephone or cable television service to providing internet connectivity, they built large and complex networks of physical hardware, from routers to switches, that must be maintained at a high cost.
Electrical and computer engineering professor Alberto Leon-Garcia, U of T Distinguished Professor in Application Platforms and Smart Infrastructure, is leading a team of researchers who will focus on replacing purpose-built hardware with flexible software that accomplishes the same tasks. This ‘softwarization’ of network infrastructure, combined with intelligence garnered from analytics and learning, greatly reduces the costs of both maintaining and updating the system. It also makes it easier to adapt the network to new devices, such as those in the Internet of Things.
Funded by a Collaborative Research and Training Experience (CREATE) grant from NSERC, Leon-Garcia’s program in Network Softwarization includes partners at three other Canadian Universities as well as companies such as TELUS, Bell Canada and Ericsson. Through courses and a series of internships, the program will prepare a new generation of engineers to build more agile, adaptable networks that are both more functional and less expensive for consumers.
SPECIALIZED EDUCATIONAL OFFERINGS IN
DATA ANALYTICS & AI
Our Master of Engineering students can choose from a wide range of technical emphases, including Robotics & Mechatronics and Analytics. Within the Engineering Science program, undergraduates can major in Robotics or Machine Intelligence — the first program of its kind in Canada to specialize in the study, development and application of algorithms that help systems learn from data. Undergraduates in our core engineering disciplines can pursue complementary studies in Robotics & Mechatronics through multidisciplinary minors.
LATEST DATA ANALYTICS & ARTIFICIAL INTELLIGENCE STORIES
OUR INNOVATION CLUSTERS
U of T Engineering has the breadth and depth of research excellence as well as the capacity to effect global change across these key domains.
OUR EXPERTS
Find the U of T Engineering researchers with the expertise to solve your most complex challenges
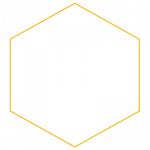
LEADING INNOVATION STARTS HERE
DOWNLOAD PDF
Connect with us to discuss how a partnership with U of T Engineering can benefit your organization.